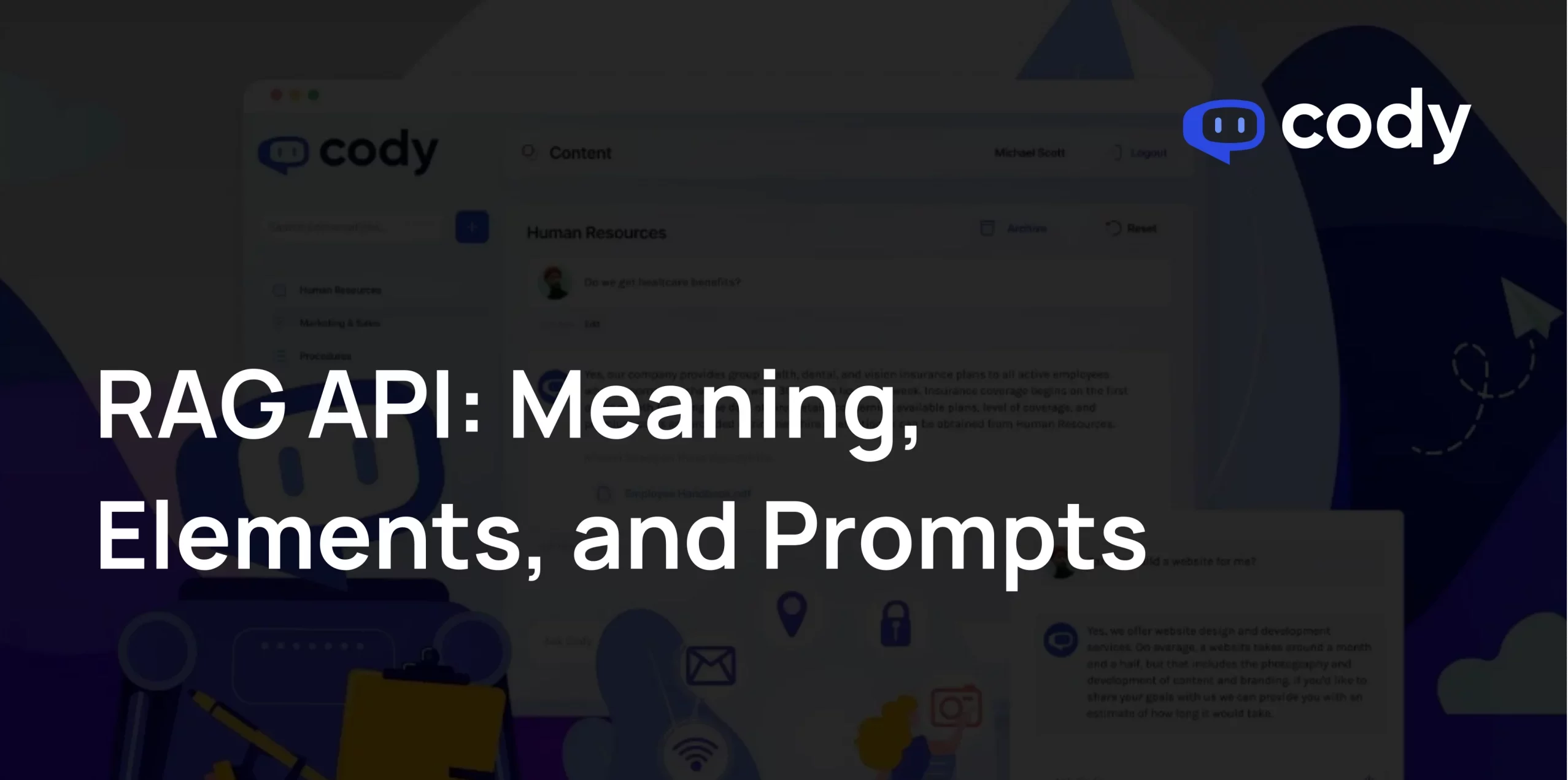
The ability to retrieve and process data efficiently has become a game-changer in today’s tech-intensive era. Let’s explore how RAG API redefines data processing. This innovative approach combines the prowess of Large Language Models (LLMs) with retrieval-based techniques to revolutionize data retrieval.
What are Large Language Models (LLMs)?
Large Language Models (LLMs) are advanced artificial intelligence systems that serve as the foundation for the Retrieval-Augmented Generation (RAG). LLMs, like the GPT (Generative Pre-trained Transformer), are highly sophisticated, language-driven AI models. They have been trained on extensive datasets and can understand and generate human-like text, making them indispensable for various applications.
In the context of the RAG API, these LLMs play a central role in enhancing data retrieval, processing, and generation, making it a versatile and powerful tool for optimizing data interactions.
Let’s simplify the concept of RAG API for you.
What is RAG?
RAG, or Retrieval-Augmented Generation, is a framework designed to optimize generative AI. Its primary goal is to ensure that the responses generated by AI are not only up-to-date and relevant to the input prompt but also accurate. This focus on accuracy is a key aspect of RAG API’s functionality. It is a groundbreaking way to process data using super-smart computer programs called Large Language Models (LLMs), like GPT.
These LLMs are like digital wizards that can predict what words come next in a sentence by understanding the words before them. They’ve learned from tons of text, so they can write in a way that sounds very human. With RAG, you can use these digital wizards to help you find and work with data in a customized way. It’s like having a really smart friend who knows all about data helping you!
Essentially, RAG injects data retrieved using semantic search into the query made to the LLM for reference. We will delve deeper into these terminologies further in the article.
To know more about RAG in depth, check out this comprehensive article by Cohere
RAG vs. Fine-Tuning: What’s the Difference?
Aspect | RAG API | Fine-Tuning |
---|---|---|
Approach | Augments existing LLMs with context from your database | Specializes LLM for specific tasks |
Computational Resources | Requires fewer computational resources | Demands substantial computational resources |
Data Requirements | Suitable for smaller datasets | Requires vast amounts of data |
Model Specificity | Model-agnostic; can switch models as needed | Model-specific; typically quite tedious to switch LLMs |
Domain Adaptability | Domain-agnostic, versatile across various applications | It may require adaptation for different domains |
Hallucination Reduction | Effectively reduces hallucinations | May experience more hallucinations without careful tuning |
Common Use Cases | Ideal for Question-Answer (QA) systems, various applications | Specialized tasks like medical document analysis, etc. |
The Role of Vector Database
The Vector Database is pivotal in Retrieval-Augmented Generation (RAG) and Large Language Models (LLMs). They serve as the backbone for enhancing data retrieval, context augmentation, and the overall performance of these systems. Here’s an exploration of the key role of vector databases:
Overcoming Structured Database Limitations
Traditional structured databases often fall short when used in RAG API due to their rigid and predefined nature. They struggle to handle the flexible and dynamic requirements of feeding contextual information to LLMs. Vector databases step in to address this limitation.
Efficient Storage of Data in Vector Form
Vector databases excel in storing and managing data using numerical vectors. This format allows for versatile and multidimensional data representation. These vectors can be efficiently processed, facilitating advanced data retrieval.
Data Relevance and Performance
RAG systems can quickly access and retrieve relevant contextual information by harnessing vector databases. This efficient retrieval is crucial for enhancing the speed and accuracy of LLMs generating responses.
Clustering and Multidimensional Analysis
Vectors can cluster and analyze data points in a multidimensional space. This feature is invaluable for RAG, enabling contextual data to be grouped, related, and presented coherently to LLMs. This leads to better comprehension and the generation of context-aware responses.
What is Semantic Search?
Semantic search is a cornerstone in Retrieval-Augmented Generation (RAG) API and Large Language Models (LLMs). Its significance cannot be overstated, revolutionizing how information is accessed and understood.
Beyond Traditional Database
Semantic search goes beyond the limitations of structured databases that often struggle to handle dynamic and flexible data requirements. Instead, it taps into vector databases, allowing for more versatile and adaptable data management crucial for RAG and LLMs’ success.
Multidimensional Analysis
One of the key strengths of semantic search is its ability to understand data in the form of numerical vectors. This multidimensional analysis enhances the understanding of data relationships based on context, allowing for more coherent and context-aware content generation.
Efficient Data Retrieval
Efficiency is vital in data retrieval, especially for real-time response generation in RAG API systems. Semantic search optimizes data access, significantly improving the speed and accuracy of generating responses using LLMs. It’s a versatile solution that can be adapted to various applications, from medical analysis to complex queries while reducing inaccuracies in AI-generated content.
What is RAG API?
Think of RAG API as RAG-as-a-Service. It collates all the fundamentals of a RAG system into one package making it convenient to employ a RAG system at your organisation. RAG API allows you to focus on the main elements of a RAG system and letting the API handle the rest.
What are the 3 Elements of RAG API Queries?
When we dive into the intricacies of Retrieval-Augmented Generation (RAG), we find that an RAG query can be dissected into three crucial elements: The Context, The Role, and The User Query. These components are the building blocks that power the RAG system, each playing a vital role in the content generation process.
The Context forms the foundation of an RAG API query, serving as the knowledge repository where essential information resides. Leveraging semantic search on the existing knowledge base data allows for a dynamic context relevant to the user query.
The Role defines the RAG system’s purpose, directing it to perform specific tasks. It guides the model in generating content tailored to requirements, offering explanations, answering queries, or summarizing information.
The User Query is the user’s input, signaling the start of the RAG process. It represents the user’s interaction with the system and communicates their information needs.
The data retrieval process within RAG API is made efficient by semantic search. This approach allows multidimensional data analysis, improving our understanding of data relationships based on context. In a nutshell, grasping the anatomy of RAG queries and data retrieval via semantic search empowers us to unlock the potential of this technology, facilitating efficient knowledge access and context-aware content generation.
How to Improve Relevance with Prompts?
Prompt engineering is pivotal in steering the Large Language Models (LLMs) within RAG to generate contextually relevant responses to a specific domain.
While the ability of Retrieval-Augmented Generation (RAG) to leverage context is a formidable capability, providing context alone isn’t always sufficient for ensuring high-quality responses. This is where the concept of prompts steps in.
A well-crafted prompt serves as a road map for the LLM, directing it toward the desired response. It typically includes the following elements:
Unlocking Contextual Relevance
Retrieval-augmented generation (RAG) is a powerful tool for leveraging context. However, the mere context may not suffice to ensure high-quality responses. This is where prompts are crucial in steering Large Language Models (LLMs) within RAG to generate responses that align with specific domains.
Roadmap to Build a Bot Role for Your Use Case
A well-structured prompt acts as a roadmap, directing LLMs toward the desired responses. It typically consists of various elements:
Bot’s Identity
By mentioning the bot’s name, you establish its identity within the interaction, making the conversation more personal.
Task Definition
Clearly defining the task or function that LLM should perform ensures it meets the user’s needs, whether providing information, answering questions, or any other specific task.
Tone Specification
Specifying the desired tone or style of response sets the right mood for the interaction, whether formal, friendly, or informative.
Miscellaneous Instructions
This category can encompass a range of directives, including adding links and images, providing greetings, or collecting specific data.
Crafting Contextual Relevance
Crafting prompts thoughtfully is a strategic approach to ensure that the synergy between RAG and LLMs results in responses that are contextually aware and highly pertinent to the user’s requirements, enhancing the overall user experience.
Why Choose Cody’s RAG API?
Now that we’ve unraveled the significance of RAG and its core components let us introduce Cody as the ultimate partner for making RAG a reality. Cody offers a comprehensive RAG API that combines all the essential elements required for efficient data retrieval and processing, making it the top choice for your RAG journey.
Model Agnostic
No need to worry about switching models to stay up-to-date with the latest AI trends. With Cody’s RAG API, you can easily switch between large language models on-the-fly at no additional cost.
Unmatched Versatility
Cody’s RAG API showcases remarkable versatility, efficiently handling various file formats and recognizing textual hierarchies for optimal data organization.
Custom Chunking Algorithm
Its standout feature lies in its advanced chunking algorithms, enabling comprehensive data segmentation, including metadata, ensuring superior data management.
Speed Beyond Compare
It ensures lightning-fast data retrieval at scale with a linear query time, regardless of the number of indexes. It guarantees prompt results for your data needs.
Seamless Integration and Support
Cody offers seamless integration with popular platforms and comprehensive support, enhancing your RAG experience and solidifying its position as the top choice for efficient data retrieval and processing. It ensures an intuitive user interface that requires zero technical expertise, making it accessible and user-friendly for individuals of all skill levels, further streamlining the data retrieval and processing experience.
RAG API Features that Elevate Data Interactions
In our exploration of Retrieval-Augmented Generation (RAG), we’ve discovered a versatile solution that integrates Large Language Models (LLMs) with semantic search, vector databases, and prompts to enhance data retrieval and processing.
RAG, being model-agnostic and domain-agnostic, holds immense promise across diverse applications. Cody’s RAG API elevates this promise by offering features like flexible file handling, advanced chunking, rapid data retrieval, and seamless integrations. This combination is poised to revolutionize data engagement.
Are you ready to embrace this data transformation? Redefine your data interactions and explore a new era in data processing with Cody AI.
FAQs
1. What’s the Difference Between RAG and Large Language Models (LLMs)?
RAG API (Retrieval-Augmented Generation API) and LLMs (Large Language Models) work in tandem.
RAG API is an application programming interface that combines two critical elements: a retrieval mechanism and a generative language model (LLM). Its primary purpose is to enhance data retrieval and content generation, strongly focusing on context-aware responses. RAG API is often applied to specific tasks, such as question-answering, content generation, and text summarization. It’s designed to bring forth contextually relevant responses to user queries.
LLMs (Large Language Models), on the other hand, constitute a broader category of language models like GPT (Generative Pre-trained Transformer). These models are pre-trained on extensive datasets, enabling them to generate human-like text for various natural language processing tasks. While they can handle retrieval and generation, their versatility extends to various applications, including translation, sentiment analysis, text classification, and more.
In essence, RAG API is a specialized tool that combines retrieval and generation for context-aware responses in specific applications. LLMs, in contrast, are foundational language models that serve as the basis for various natural language processing tasks, offering a more extensive range of potential applications beyond just retrieval and generation.
2. RAG and LLMs – What is Better and Why?
The choice between RAG API and LLMs depends on your specific needs and the nature of the task you are aiming to accomplish. Here’s a breakdown of considerations to help you determine which is better for your situation:
Choose RAG API If:
You Need Context-Aware Responses
RAG API excels at providing contextually relevant responses. If your task involves answering questions, summarizing content, or generating context-specific responses, RAG API is a suitable choice.
You Have Specific Use Cases
If your application or service has well-defined use cases that require context-aware content, RAG API may be a better fit. It is purpose-built for applications where the context plays a crucial role.
You Need Fine-Tuned Control
RAG API allows for fine-tuning and customization, which can be advantageous if you have specific requirements or constraints for your project.
Choose LLMs If:
You Require Versatility
LLMs, like GPT models, are highly versatile and can handle a wide array of natural language processing tasks. If your needs span across multiple applications, LLMs offer flexibility.
You Want to Build Custom Solutions
You can build custom natural language processing solutions and fine-tune them for your specific use case or integrate them into your existing workflows.
You Need Pre-trained Language Understanding
LLMs come pre-trained on vast datasets, which means they have a strong language understanding out of the box. If you need to work with large volumes of unstructured text data, LLMs can be a valuable asset.
3. Why are LLMs, Like GPT Models, So Popular in Natural Language Processing?
LLMs have garnered widespread attention due to their exceptional performance across various language tasks. LLMs are trained on large datasets. As a result, they can comprehend and produce coherent, contextually relevant, and grammatically correct text by understanding the nuances of any language. Additionally, the accessibility of pre-trained LLMs has made AI-powered natural language understanding and generation accessible to a broader audience.
4. What Are Some Typical Applications of LLMs?
LLMs find applications across a broad spectrum of language tasks, including:
Natural Language Understanding
LLMs excel in tasks such as sentiment analysis, named entity recognition, and question answering. Their robust language comprehension capabilities make them valuable for extracting insights from text data.
Text Generation
They can generate human-like text for applications like chatbots and content generation, delivering coherent and contextually relevant responses.
Machine Translation
They have significantly enhanced the quality of machine translation. They can translate text between languages with a remarkable level of accuracy and fluency.
Content Summarization
They are proficient in generating concise summaries of lengthy documents or transcripts, providing an efficient way to distill essential information from extensive content.
5. How Can LLMs Be Kept Current with Fresh Data and Evolving Tasks?
Ensuring that LLMs remain current and effective is crucial. Several strategies are employed to keep them updated with new data and evolving tasks:
Data Augmentation
Continuous data augmentation is essential to prevent performance degradation resulting from outdated information. Augmenting the data store with new, relevant information helps the model maintain its accuracy and relevance.
Retraining
Periodic retraining of LLMs with new data is a common practice. Fine-tuning the model on recent data ensures that it adapts to changing trends and remains up-to-date.
Active Learning
Implementing active learning techniques is another approach. This involves identifying instances where the model is uncertain or likely to make errors and collecting annotations for these instances. These annotations help refine the model’s performance and maintain its accuracy.