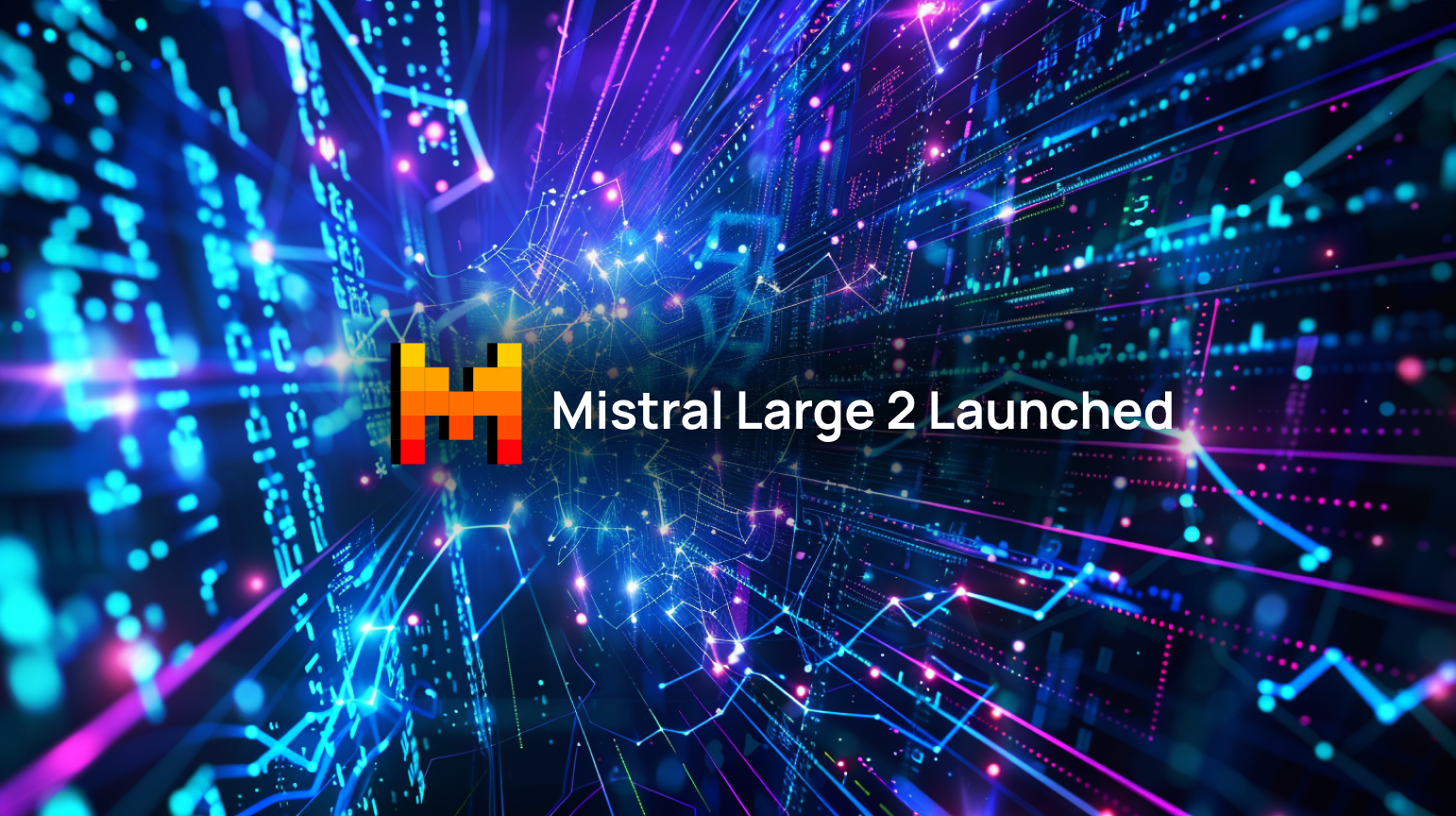
Mistral Large 2 features an impressive 128K context window and supports dozens of languages, including major ones like English, French, German, and Chinese, as well as more specific languages such as Hindi and Korean. Additionally, it supports over 80 coding languages, making it an indispensable resource in our increasingly globalized world .
The model is also designed with cost efficiency in mind, allowing for both research and commercial usage. This balance of high performance and affordability positions Mistral Large 2 as a highly competitive option in the AI landscape .
Key Features of Mistral Large 2
Mistral Large 2 boasts a 128K context window, significantly enhancing its ability to process extensive and complex datasets. This vast context window expands the model’s capability to understand and generate relevant responses across varied contexts.
The model supports dozens of languages, covering major global languages such as English, French, German, and Chinese. Additionally, it includes more specific languages like Hindi and Korean, making it invaluable for diverse linguistic applications.
Besides, Mistral Large 2 excels in coding, offering support for over 80 programming languages, including Python, Java, and C++. This feature makes it an ideal choice for developers working on complex coding projects.
With 123 billion parameters, the model enhances reasoning capabilities, ensuring more accurate and reliable outputs. A particular focus was placed on minimizing AI-generated hallucinations, thereby improving the model’s reliability in delivering precise information. For more insights into the benefits and risks of large language models, you can explore this article on Open Source Language Models.
Performance and Cost Efficiency
Mistral Large 2 achieves an impressive 84.0% accuracy on the MMLU benchmark, positioning it favorably against other models in terms of performance and cost efficiency. This high accuracy underscores the model’s ability to provide reliable and precise outputs, making it a strong contender among leading AI models.
The model’s performance/cost ratio is noteworthy, placing it on the Pareto front of open models. This indicates that Mistral Large 2 offers a balanced combination of performance and cost, making it an attractive option for both developers and enterprises.
Additionally, Mistral Large 2 is available under two licensing options: a research license that allows usage and modification for research and non-commercial purposes, and a commercial license for self-deployment in commercial applications.
When compared to rival models like GPT-4 and Llama 3, Mistral Large 2 demonstrates competitive performance, particularly in handling complex tasks and delivering accurate results in various applications.
Integration and Accessibility
Mistral AI models, including Mistral Large 2 and Mistral Nemo, are designed for seamless integration and accessibility across various platforms. These models are hosted on la Plateforme and HuggingFace, making them easily accessible for developers and enterprises alike.
Additionally, Mistral AI has expanded its reach by ensuring availability on leading cloud platforms such as Google Cloud, Azure AI Studio, Amazon Bedrock, and IBM watsonx.ai. This broad accessibility supports a variety of development and deployment needs.
A notable collaboration with Nvidia for the Mistral Nemo model further enhances the models’ integration capabilities. Mistral Nemo, with its state-of-the-art features, is a powerful drop-in replacement for systems currently using Mistral 7B.
Azure AI provides an added layer of enhanced security and data privacy, making it an ideal platform for deploying these robust AI models. This ensures that sensitive data is well-protected, meeting enterprise-grade security standards.
Mistral AI – Leading the Future of Advanced AI Solutions
Mistral Large 2 and Mistral Nemo are at the forefront of AI innovation, offering unparalleled performance, multilingual proficiency, and advanced coding capabilities. Mistral Large 2’s 128K context window and support for over a dozen languages, combined with its superior reasoning and coding potential, make it a standout choice for developers aiming to build sophisticated AI applications.
The models’ broad accessibility through platforms like la Plateforme, HuggingFace, and leading cloud services such as Google Cloud, Azure AI, Amazon Bedrock, and IBM watsonx.ai ensures that enterprises can seamlessly integrate these powerful tools into their workflows. The collaboration with Nvidia further enhances the integration capabilities of Mistral Nemo, making it a robust option for upgrading systems currently using Mistral 7B.
In conclusion, Mistral AI’s latest offerings provide a significant leap forward in the AI landscape, positioning themselves as essential tools for next-generation AI development.