Semantic Search vs. Fine-Tuning: Which is Best for Training AI in Your Business?
In today’s technologically-driven business landscape, leveraging artificial intelligence effectively is paramount. With the rise of advanced models like GPT-3.5, businesses are often faced with a crucial decision: Should they fine-tune these models on their specific datasets, or should they pivot towards semantic search for their requirements? This blog post aims to shed light on both methods, providing a comprehensive comparison to help businesses make an informed decision.
Understanding Fine-Tuning
Fine-tuning is analogous to refining a skill set rather than learning an entirely new one. Imagine a pianist trained in classical music; while they have a foundational understanding of the piano, playing jazz might require some adjustments. Similarly, fine-tuning allows pre-trained AI models, already equipped with a wealth of knowledge, to be ‘tweaked’ for specific tasks.
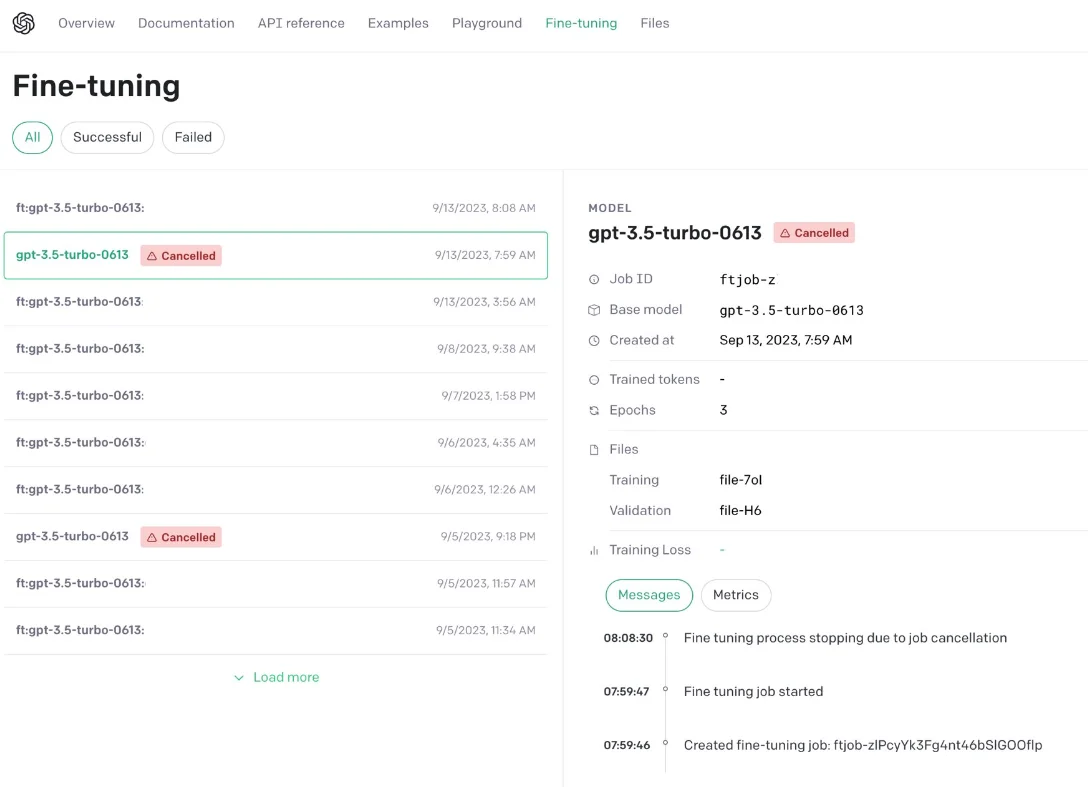
In the realm of AI, fine-tuning is an application of transfer learning. Transfer learning allows a model, trained initially on a vast dataset, to be retrained (or ‘fine-tuned’) on a smaller, specific dataset. The primary advantage is that one doesn’t start from scratch. The model leverages its extensive prior training and adjusts its parameters minimally to align with the new data, making the learning process quicker and more tailored.
However, a common misconception is that fine-tuning equips the model with new knowledge. In reality, fine-tuning adjusts the model to a new task, not new information. Think of it as tweaking a guitar’s strings for optimal sound during a performance.
Demystifying Semantic Search
Semantic search is a revolutionary approach that takes searching a notch higher. Traditional search methods rely on keywords, returning results based purely on word matches. Semantic search, on the other hand, delves deeper by understanding the context and intent behind a query.
At the heart of semantic search are semantic embeddings. These are numerical representations that capture the essence and meaning of textual data. When you search using semantic search, you’re not just matching keywords; you’re matching meanings. It’s the difference between searching for ‘apple’ the fruit and ‘Apple’ the tech company.
In essence, semantic search offers a more intuitive, context-aware method of retrieving information. It understands nuances, making it immensely powerful in delivering precise and relevant search results.
The Fine-Tuning vs. Semantic Search Showdown
When weighing fine-tuning against semantic search, it’s essential to recognize that they serve different purposes:
Criteria | Fine-Tuning | Semantic Search |
---|---|---|
Purpose & Application | Aimed at task optimization. For instance, if a business has an AI model that understands legal language but wants it to specialize in environmental laws, fine-tuning would be the route. | The objective is information retrieval based on meaning. For example, if a medical researcher is looking for articles related to a specific type of rare disease symptom, semantic search would provide deep understanding results. |
Cost & Efficiency | Can be resource-intensive both in terms of time and computational power. Each addition of new data might require retraining, adding to the costs. | Once set up, semantic search systems can be incredibly efficient. They scale well, and incorporating new data to the search index is generally straightforward and cost-effective. |
Output | Produces a model better suited to a specific task. However, fine-tuning doesn’t inherently enhance the model’s knowledge base. | Yields a list of search results ranked by relevance based on a deep understanding of content. |
Final Thoughts
Recalling the age-old practice of searching for the right book in libraries using the Dewey Decimal System, skimming pages, and compiling notes to derive answers serves as a metaphor for how AI processes information.
In this digital age, where data is the new oil, the decision between fine-tuning and semantic search becomes pivotal. Each method has its strengths, and depending on specific needs, one might be more suitable than the other, or even a blend of both.
As businesses increasingly look to optimize processes and enhance efficiency, tools like Cody that can be trained on specific business processes become invaluable assets. And for those eager to experience this AI transformation, the barrier to entry is virtually non-existent. Cody AI offers businesses the chance to start for free, allowing them to harness the power of semantic search without any initial investment. In the ever-evolving world of AI and search, Cody stands as a testament to the potential of semantic search in revolutionizing business operations.